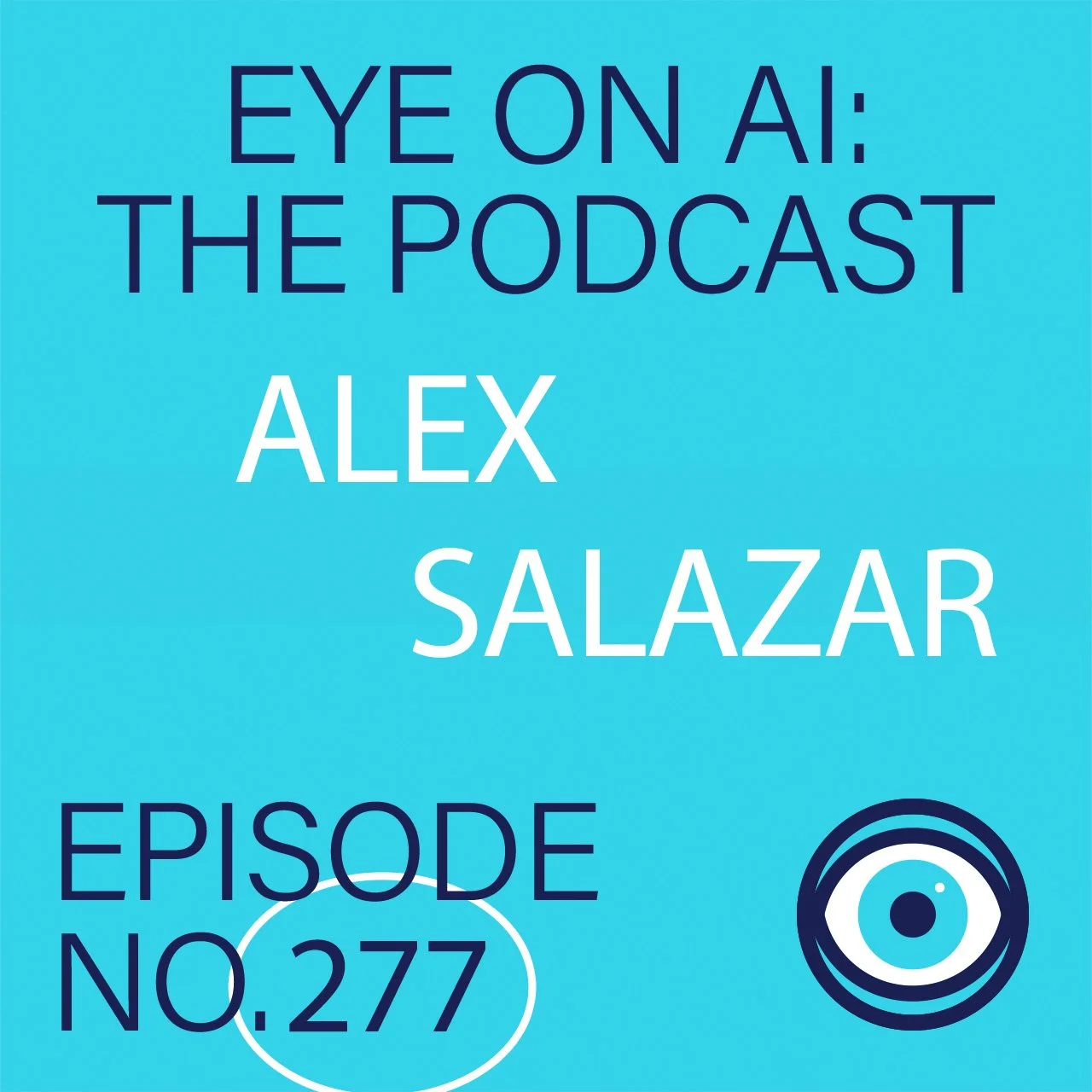
Alex Salazar, co-founder and CEO of Arcade.dev, will discuss the requirements for building secure, scalable AI agents capable of real-world actions.
277 Audio.mp3: Audio automatically transcribed by Sonix
277 Audio.mp3: this mp3 audio file was automatically transcribed by Sonix with the best speech-to-text algorithms. This transcript may contain errors.
Alex:
In this one prompt, I've asked it to do three different things. What's also important about this prompt that we're about to execute is that all of these things I'm asking it to do are ultimately on other third-party services. Right, we're going to talk to Gmail, we're going to talk to Slack, we're going to talk to GitHub and that itself is pretty difficult for agents but, most importantly, all of these services are secured. You can't access someone's Gmail unless you've authenticated as them, and similarly for Slack. So all of this is happening on behalf of me. People are learning the hard way that APIs don't work with large language model very well. You can't just hand a large language model, an API definition, and have it go figure it out. It might, but the error rates, hallucinations, it won't work with enough confidence to make it valuable.
Craig:
Our sponsor for this episode is Xtreme Networks, the company radically improving customer experiences with AI-powered automation for networking. Xtreme is driving the convergence of AI, networking and security to transform the way businesses connect and protect their networks, to deliver faster performance, stronger security and a seamless user experience. Visit XtremeNetworkscom to learn more. That's XtremeNetworks all run togethercom to learn more.
Alex:
That's extremenetworksallruntogethercom to learn more. So, hi, I'm Alex Salazar. I'm the founder CEO of arcadedev and, at a high level, we make it possible for AI agents and large language modelbased applications to perform actions and connect to other systems and other services securely. And to put it in a very relatable way, most of us have used ChatTPT to draft an email, but you can't send the email from ChatTPT, and that's because chat TPT agent can't properly connect to Gmail and authenticate as you in order to send the email or read the emails, and vice versa. So we help solve that. Um.
Alex:
Prior to starting the company, um, I did a brief stint in venture capital, um at a deep tech venture firm called Neo tribe. Um. That's where I ran into the problem. I was mostly focused on AI infrastructure and started to see where the challenges were in AI and, as a former founder sitting in venture capital, I couldn't help but get the itch and go start the company that I wanted, instead of funding it. Prior to that, I was VP of product for Okta for their developer products and the home manager for all their new products, and prior to that, I was a CEO co-founder of a company called StormPath, which was an API for developers to implement authentication login password reset in their applications, and Okta acquired that business. That's part of its developer-facing customer identity business. So my background is heavily in authentication, authorization, developer products and helping organizations change how they do business with their customers through software. And then a computer science degree from Georgia Tech and an MBA from Stanford University.
Craig:
Okay, and the arcadedev is? How would you describe it? It's a platform for building agents.
Alex:
Yeah, Correct. So our customers are developers, people trying to build agents themselves, so typically organizations or, or you know, all the way down to hobbyists who are trying to build something fun for themselves.
Craig:
But it's our customers are building the agents and then we're giving them the ability to connect those agents securely to other services like Gmail and Slack and Twitter and Salesforcecom and anything that might be custom and internal to their organization talking at some point about and this may have been the way that we met, I don't remember about a model context protocol developed by Anthropic and I know you guys have done some work with Anthropic on developing that protocol. And I was just at a conference with IBM where they were introducing their agent communication protocol, acp, and all of that sounds like it would fit in the Arcadedev platform. So, yeah, can you talk through how a developer would use arcade uh and whether IBM's uh ACP and Anthropix MCP are are the dominant protocols or whether there are a bunch of them?
Alex:
Yeah, yeah, it's man. It's a great question, um, so I'll start with the protocols. Uh, there are. There is an exploding number of protocols right now, but they're all in their infancy, it is like the very beginning of the first inning. And so I would say MCP is the one that's most talked about and it is getting tremendous popularity because some of the coding agents that developers use to write software the most popular one being Cursor adopted MCP and that really exploded its popularity with developers when people are building agents.
Alex:
There's a lot of promise with these protocols. There's a lot of promise with these protocols. There's a lot of promise with MCP. In fact, we're pretty heavily involved with Anthropic in helping to evolve MCP properly, specifically around authentication and authorization, but it's still very early. But the protocols are really about helping everybody agree on how an agent and another service are going to are going to talk to each other. That just like you know that language between the two Um. So we as a, as a, as a platform that helps developers build this and automate these connections and and properly handle the authentication and authorization and the retry logic and all that, we're ultimately protocol agnostic. We believe in MCP and we're investing heavily in MCP, but I don't think that developers ultimately care at that level. What they really care about is they want to make sure it all works Um, and so that's where we put all of our energy is just making sure developers are able to check the box, deliver the product they want to deliver, and and we'll give it to them in the best way we can.
Craig:
And, um, yeah, so let's go to the platform and and you can introduce the platform and what it does. And I'm guessing, with these protocols is there a drop-down menu and you pick the protocol. I mean, how does that?
Alex:
work? Yeah, it's a great question, so I'll give a quick demo. I'll give a quick demo and, for people listening, I'll walk you through it and we can all experience it in the theater of the mind. All right, so what I'm showing here is not actually our product. It's what we refer to as a sample application and that's an example of what a developer could build using our product and the kinds of features that they could deliver.
Alex:
And so, for the people who can't see the screen, this looks very much like a chat TPT type interface. It looks like a chat bot, and so one of the big differences is, at the very bottom you're going to see this little dropdown that says toolkits. This is our terminology for all the connectors and plugins to other services that you might, that you might have in your agent, and so we've set up five toolkits here. We've got GitHub, uh, google workspace, so like things like Gmail, uh, linkedin, uh, notion and Slack, and of those toolkits themselves are going to have a set of what people in AI refer to as tools. So each of them will have let's call it on average about 10 tools. The tools themselves are the actions, and so if it said, hey, read my email. Reading an email is an action. Reply to the email, that's another action. Send the emails another action. And so each of these tools have at least 10, the Google one's actually pretty beefy that one has a lot more than 10. And and so that's going to dictate what the agent can do. So let me show you a use case. So we're going to say, read my, read the general channel on Slack, pull the latest activity on the arcade AI, arcade AI, github repo and send a summary of it all to Alex at arcadedev.
Alex:
So what I've written but not yet submitted is what's called a multi-turn prompt. And so in Chat2BT, you'll typically say, hey, write me a poem, and that's one turn. But if you say, hey, write me an email and then summarize it, and then so the thing, the LLM, the agent using LLM, will have to take multiple turns to achieve what you want. And so in this one prompt, I've asked you to do three different things. What's also important about this prompt that we're about to execute is that all of these, you know, all of these things I'm asking you to do, are ultimately on other third-party services. Right, we're going to talk to Gmail, we're going to talk to Slack, we're going to talk to GitHub, we're going to talk to Slack. We're going to talk to GitHub, and that itself is pretty difficult for agents. But, most importantly, all of these services are secured. You can't access someone's Gmail unless you've authenticated as them, and similarly for Slack. So all of this is happening on behalf of me. This is end user-based authentication and authorization, which I haven't seen many examples of in the world today. So for many people, this is the first time the library sees something like that. So we're going to hit enter and we're going to pray to the demo gods that it works.
Alex:
Live demos are always tricky, and so what's happening is we sent the prompt to the large language model, and this is an off-the-shelf large language model. We're using GPT-4.0. And along with the prompt under the hood, we sent it a collection of like a multiple choice question of all these different tools available to it, and we sent about 50, which is a very large number. Most engineering teams can't get past 10 without the LLM hallucinating. We're able to get to about 80 or 100. So we sent about 50.
Alex:
In this scenario, and think of it like a calculator If somebody asks you to multiply two really large numbers, you're probably not going to get it right. But if I hand you a calculator, you're going to get it right. But if I hand you a calculator, you're going to get it right. And so what we've done here is all of these tools are like calculator buttons, instead of the large language model trying to figure out what it even means to look up something in Slack, it knows to hit the button that it hit here, which is the Slack get message in channel by name tool. And then, and then it also went and hit the GitHub list repository activities tool. Yeah, that's all it had to do. And then it passes us the arguments. So it needs to know, you know, hey, channel by name, which channel based on the prompt is guessing the general channel. Hey, on GitHub, which repository based on the prompt it's guessing the general channel. Hey, on GitHub, which repository based on the prompt it's hitting the arcade-ai repo. And then we execute all of that logic for the agent.
Alex:
Now, when we go receive that request to go execute those tools, the very first thing that happens in Arcade is we check to see does this tool require authentication and authorization? And in both cases the answer is yes. And so then we say, okay, great, is that user for whom the agent's acting on behalf of, has it already authenticated and authorized the agent to perform these actions? And if the answer is yes, we proceed. If the answer is no, then we pop up a login screen and the developer goes through what's called an OAuth flow, which almost all of us have experienced on the internet, where you know you go to the Google's website and there's a screen telling you hey, do you want to, like, allow this other application, all these rights to your system, yes or no? And you hit approve, we handle all of that for the developer. And then, once the user does that, it sends the alert back to the agent and the agent proceeds with the requests, which is what's happening here, and then you know. So that's how the service works.
Craig:
Yeah, let me just go back a little bit. So, when you make the initial request, the platform is like an orchestrator it knows what tools it has access to and rather than as I've seen with many platforms you deciding which tool you need to use for this workflow, it'll automatically make that decision for you. And then it's just a question of whether it has authorization, whether the platform has already gone through authorization, for example, to access your Gmail.
Alex:
Correct no-transcript. So, if you think of how security works at a really high level, there's a resource let's think your email is the resource and then there's the subject, the person trying to access the email. And so in most scenarios, as humans, it's a very simple process we show up, we show up, there's a login screen, we type in our information and then we're in, and then there's permissions inside the system saying what do we have access to which is in our Gmail? We have access to all of it, but you know, if we're trying to access, you know, the finance system at our organization, we probably have very limited access. Yeah, very simple use case.
Alex:
There's also the concept of machine identity, which is not new. This has been around forever, and so you know you might give an API key to another service, another piece of software, and then that service can go authenticate in its way, very similar way to you know another service, another piece of software, and then that service can go authenticate in its way, very similar way to you know another service, like a finance system, and might be able to pull information, and once it authenticates, it has it itself, has all kinds of permissions that it accesses. But this is different, and so the number of use cases where an AI agent can and should access something as itself and purely as itself what we call a service account are pretty limited. You know it's the Google search API, it's a weather API. It's typically things that aren't terribly secure. You know you don't worry a ton about them getting broken into, but the vast majority of where end users get value from an agent require the agent to go access something that is tied to the user. You want to access your email. It's tied to you. You want to access you know financial information in your organization. It's going to be tied to the user. You want to access your email. It's tied to you. You want to access financial information in your organization. It's going to be tied to who's reading it. The CEO is going to have a very different level of access than the intern, and so when you do that, the naive approach is to have the agent simply get the user credentials and just authenticate as the end user to those services. That's wildly insecure and, more importantly, it's unsafe from an AI perspective.
Alex:
So let's use an email example your inbox. You can do whatever you want. You can delete all the emails if you wanted to Do. You want to give the agent the ability to delete email? No, because it might hallucinate and accidentally to delete email? No, because it might hallucinate and accidentally delete your email.
Alex:
There's a very famous coding agent called cursor that I use actively, sure it. It wants, hallucinated and tried to delete my root directory. Wow right, for those people who aren't engineers, you know, deleting your root directory is the equivalent of deleting your entire computer. Now, thankfully, you know, my Mac operating system has a bunch of authorization already built in, and so even if I had said yes, it wouldn't have been able to do it, Thank God. But you don't want to. Even though you, craig, have full access to your email, you don't want to give the agent that same level of access, and so the right approach is to give the agent an intersection of permissions, an intersection of what you, craig, have access to, but also an intersection of what the developer and Craig have granted the agent access to, and that, thankfully, there's protocols for that. There's a very popular protocol called OAuth, but it's very difficult for developers to implement and it's, until recently, almost impossible for them to implement in the context of agents, and that's what we've solved.
Craig:
I see, and in that case the well, let's go back to the API issue, because LLMs don't handle APIs very well. What happens when you need to hit a service or yeah, that's a great question.
Alex:
So I think this is the thing that a lot of people are are learning. I mean, the ai industry is very new, right? Uh? You know chat, tpt and gpt 3.5, you know, had their second year anniversary this last november, right it right, and so it's. It's mind-boggling to to really like internalize how fast this all happened. Um, and so people are, as people are trying to build agents that can take, that can take actions, that can do things and not just generate content.
Alex:
People are learning the hard way that apis don't work with large language model very well. You can't just hand a large language model an api definition and have it go figure it out. It might, but the error rates, what people you know hallucinations will make it just not very like won't work with enough confidence to make it valuable, and so what people do instead the right way to give an agent access to other services is to build what's called a tool Terrible name, seo is terribly, but it's the terminology everyone in AI uses and a tool is like an action. It's an intent-based action that might wrap one, zero, one or multiple APIs to perform that action. And so I'll give you a really simple example Reply to an email.
Alex:
If you're in an agent that's email-based and you say, hey, reply to my email from Craig. There is no API endpoint on the Gmail API to respond to an email. There is lookup email and there is send email and so a reply to Craig. You know, when I say reply to Craig's email, the tool it needs is going to be reply to email tool. That tool is going to do multiple things. The first thing it's going to do is it's going to go find the email I'm referencing that I want it to reply to. So that's already one API call.
Alex:
Once it retrieves that email, it then has to unpack the email structure. It's called MIME. You have to unpack this email structure. It's actually a fairly complex piece of software. There's attachments, there's HTML versions and full text versions etc. Unpacks all of that, then has to go generate the response, insert the response, then repackage it all up again in that email structure called MIME and then hit the send email endpoint. Altogether it's about 100 or 200 lines of code, depending on how diligent you're being, and that's not even including all the error handling and checks to make sure it's all working and safe. That's a lot of work.
Alex:
That's a distinction between an API and a tool. Now you go something much more complicated like hey, doordash, order me a pizza. The order me food tool for DoorDash, order me a pizza, the order me food tool for DoorDash would be like 10 API calls. So there's just a different level of complexity. But, most importantly, it's presented to the large language model very differently. If you've ever looked at an API, it looks like gibberish, it looks like a URL. Ai systems don't understand that. So a large language model, when the tools presented to it, it's presented in natural language. English is a tool for replying to an email by a sender. It takes in the name of the sender, the subject line, the body of the new response and it's all explained in English. And that's what makes a letters language model really good at selecting the right tool and then selecting the right input arguments for it to go execute.
Craig:
Okay, and and the um, this all takes place internally. I mean, is that the chief because there are a lot of platforms out there now to build agents a differentiator with Arcade that it handles this authorization in the backgrounds, but you don't have to.
Alex:
Yeah, so the way to describe where we fit.
Alex:
so we are not a large language model company yeah they're very good large language model companies out there, anthropic and open ai being the most notable and so the large language model is the one that selects the tools. We we've designed arcade to be the system that receives the selection and then executes the tool, and so what we're really good at, at a really high level, is helping developers get that tool execution to be so good that they can get their agent from a demo to production, and so anything that requires that we've put a lot of IP into. The very biggest problem everybody runs into is that end user authentication and authorization, and so that is the backbone of our feature set. But there are other things that are also critical, but they're a bit in the weeds, like parallel tool calling, retrieval tool logic. So that demo I gave you. It works remarkably consistently, which in AI, that is the performance metric.
Alex:
In AI it's not how fast it is, it's how often does it error out? That demo I gave you. I've given that demo like 100 times. I don't think I've ever seen it error out, and it's not because the large language model is not hallucinating. It is hallucinating just like every other system out there. But part of our ip is that we can catch those errors and then have the large language model retry with additional context, and when you do that, the consistency rates go through the roof. And so all of that nitty gritty detail that helps a developer take an agent from demo to production, that's where we focus our efforts.
Craig:
Yeah, and this is a developer's platform, why not make it a B2C product for general users?
Alex:
Yeah it's a great question To know thyself right. My team and I, we're just passionate about infrastructure. Yes, I always give a house analogy. I'm trying to give you the best pipes in the industry. I want you to build a beautiful house, Right? Um, I, I just happen to be really passionate about pipes.
Craig:
And yeah, but that doesn't explain why not let you know mom and pop build their own.
Alex:
You know, I think from a consumer side, oh, why not let consumers use the service to build their own agents? Yeah, okay, that's different. Yeah, so the answer I was giving why didn't we just build an application Like why didn't we compete with Chatsy, right, right, oh, I understand that. So, there we want to be, you know, be picks and shovels. Why do we not expose our picks and shovels to the average consumer?
Alex:
Um, there, you know, people call this like, you know, no code or low code agent builders. Um, that's not a space one. We don't know that space particularly well. So there is a bit of, like a domain mismatch, um, but second, I, if I'm being perfect honest, I'm just not a big believer, um, in those, um, because it's very the building agents extraordinarily complicated and, um, you know, we really, we really want to have a big impact on what everybody in the world, what the world of AI and agents looks like, and I think that the biggest impact we could have would be giving it to the developers, because they're the ones who, I think, are going to, we believe they're the ones who are going to build the most powerful agents of tomorrow, tomorrow, and so that's that's where we focused yeah, and so on the platform, once you've built an agent, uh, how is it deployed or how is it moved into a production?
Craig:
uh, product.
Alex:
Yeah, so, um, the the kind of the life cycle of of an agent developer is uh, they start to build something and they get working in demo and everybody gets wowed. Um, you know, I'm sure you know, you've seen a lot of agents wow you in a demo and then, yeah, you know, they never quite make it to production. It's it's actually one of the biggest problems right now in agents is, I think, like the stat I heard somewhere is less than 30 percent of agents ever make their way to production. Uh, because it's so hard. The demos are easy, and so what happens next is they show it to everybody, they get approval to go really invest in it, and then they start to build and start running into a bunch of problems.
Alex:
The biggest problem they run into, the first one they run into, is authentication and authorization, because they simply can't deliver a feature. Let's say they get past that. The next problem they run into is consistency and accuracy. Right, notoriously, agents and AI hallucinate, and if those hallucination rates are below 80%, it's not going to be a valuable agent, people aren't going to use it, and so a lot of things fail over that. Now, if you clear that hurdle, the next thing you're going to run into is a cost issue Open AI and Anthropic they cost money Every time you call them. You're paying for each token and that stuff can really add up at scale.
Alex:
So a lot of teams that we talked to who don't go to production yet. Somebody did math and realized, oh, if we implement this, it's going to bankrupt us, and so then there's some re-engineering of how, if we implement this, it's going to bankrupt us, and so then there's some reengineering of how do we implement this with fewer large language model calls. Now, if you're using us, we unblock the first two. The third one is fortunately out of our hands, and so to deploy us it's very easy.
Alex:
The developer gets an API key from us, drops it into their software and when they're implementing their logic, they're just calling out to us for what's called the tool calls and then we handle everything else behind the scenes. So we've offloaded a lot of the logic so that they said to drop an API key and that's typically it for them. In most use cases. If a developer wants to build their own integration, they want to author their own tool. We have a whole SDK for them to do that and leverage all the same value and benefits, and how they deploy that custom tool really depends on what they want to do. They can deploy it in their own infrastructure, their own servers, or they can deploy it to us and then we'll put it on a cloud server and run it for them, but that's really all that's needed yeah, and then does it create a web app for the tool that then the developer can disseminate within their organization or um, how so?
Craig:
I'm a developer at a large organization. I, I create this uh agent to help people manage their emails, read and send, and and all of that uh, that the the actual um logic of that, that the the actual logic sits in in the cloud with arcade. I hit it with an API, but what's the interface that?
Alex:
John Greenewald that's a great question. So this important distinction. So the agent itself, let's call it. Let's call it the app, because an agent is just an application. The difference is that it's leveraging large language models for the workflows. So instead of a developer somewhere saying, okay, step one, step two, step three, in this order, there's a large language model in there and the large language model is deciding what the next step in the workflows are, whether whether it's a consumer facing you know, uh, writing app, or a crm or a finance app.
Alex:
That's a distinction. That app we don't touch, um, that app, that that app's deployed and written in whatever way the developers want. So so you know, maybe it's deploying to AWS or to Microsoft Azure, but when it goes to take an action that talks to another service, like an integration, at that point that app calls out to us Right, and for most of our customers we are used as a cloud service ourselves, but for our larger customers they can deploy us in their own private you know private clouds, their own, you know their own private Azure's and AWS environments you say you're not a model company, then you can choose the model based on whatever, whether it's price or latency or whatever.
Craig:
I was just up to see IBM's rollout of Granite 4, and they're building these small models that are much cheaper and for something like reading and responding to an email, you don't need a behemoth like 4.0. Do you have smaller models like that?
Alex:
yeah, so for us in the demo I gave you that demo demo uses GPT-4.0, but that's really just for the demo. For our customers they can bring whatever models they want. Now, that being said, when it comes to tool calling the ability for a large language model to decide and select that right calculator button to hit and what the input arguments are, the calculator button today there are very few models that can do tool selection, or what's called tool calling or function calling, right today that I know of, I think it's just um, open, ai, openai, gpt 3.5, turbo and Up Clod. I know that Meta's Llama, the most recent Llama release, now supports tool calling and I think Grok. So the population of the ones that can actually integrate the tools is still pretty small. That's going to change quickly. But yes, we're believers. Small, that's going to change quickly. But um, but yes, we're, you know, we're believers that there there are going to be a proliferation of smaller models for more specific tasks, more narrow tasks, but uh, but they're not yet tool calling capable.
Craig:
They'll come yeah, yeah, um, and and this, how you price arcade, is it a subscription by seat or pay as you go?
Alex:
usage model developers love Pay as you go. We do have subscriptions, but they're really just proxies for usage-based pricing to make it simpler, and so we charge on two metrics. We charge on monthly active users that we're authenticating and authorizing, because the identity component of the product is very important, and then, separately, we charge on what we call request volume how often are you calling out to our service to perform an action? Both of those metrics we see as metrics of the value that someone is getting from our service.
Craig:
Yeah, and how. I mean, this is all moving so fast and, as I said, there are a lot of these platforms for building agents. Two questions how do other platforms handle this authentication issue yeah, issue and how do you compete with the likes of IBM, who has an agent building platform or AWS, or all these people are? Coming out with agent building platforms.
Alex:
Yeah, so for the smart agent building platforms like relevance, ai, they use us, right, you know, we are the connectors. You know, if you look at one of the more popular ones is, you know, n8n, a very robust community. If you look at how the connections work, they don't do this. You have to really do abnormal things. To connect to Google Drive, for example, it really only works for one user because it's using that particular user's credentials hard-coded in to the agent. That's the state of the art today For anyone building an agent builder.
Alex:
We make all of this very easy, uh, and very secure, uh, without them having to go spend, you know, you know, a year of engineering time trying to figure out how to make it work. Half our team is out of Okta. We nailed the office, um. We don't compete with auth builders, um and so, because our target demographic, the software developers, they're not really using agent builders, right, what they're writing the agents themselves in code, because they, they need that bespoke, you know, fidelity of how it's going to work. Morgan stanley's not using ancient builder, right, um and so. So we partner very closely with what what they're most likely using, which is, which are called orchestration systems. So, like a lang chain is a really good example. So there we partner very closely with them. They handle the orchestration and we handle the tool column.
Craig:
Yeah, that's interesting, and where do you see this going? I mean, um, you know people are now talking about, you know, these networks. Microsoft calls it a society of agents, or societies of agents that will be operating alongside human workers. I mean, how do you see that developing and do you see that in your usage metrics?
Alex:
I'm a very big believer in agents. I'm an agent maximalist, if you will, but I do think that sometimes the PR gets a little too far out from some of the reality. So here's what I believe out from from from some of the reality, um, so here's here's. Here's what I believe. Um, I believe that what's happening with agents is very similar to what's happened with the internet in 94, right, um, uh, open AI, uh and anthropic are the Netscape navigator of this moment and they, like, unlocked all of this innovation, and so now we're seeing it all happening in real time. It's happening very, very and I think, by all metrics, happening about twice as fast and twice as big as the internet boom, but, and so I think it's going to radically change our lives in every way.
Alex:
Right, I look at my kids. Already my 14-year-old daughter gets upset when she sees me typing, calls me a dinosaur, yanks the phone from my hands, hits the dictate button and talks to the phone. I use my Android and I won't let go of it because the AI assistant is so far superior to Siri and only going to get better once the gemini models officially hit the assistant level, um, and so I no longer interact with my phone. You know as much. With my thumbs right I talk to it and so all of like that natural language interface is super powerful because it captures intent. The best business in history was Google and it had one input box. The entire business model was one input box and it was so powerful because it captured user intent.
Alex:
If you go look at something like Salesforcecom, man, you've got to go through three months of software of training just to use a damn thing because it's so complicated, got to go through three months of software of training just to use a damn thing because it's so complicated. All of that's going to go away when you can just say, hey, give me the latest user record from you know for my meeting with craig. Nope, that's the where all this stuff is going. I, I believe, but I don't. I, I, I typically. I personally bristle when people personify agents because I think in five years the conversation is going to be really boring, like it's going to be so powerful and so valuable. But it's also going to look really boring in hindsight, just like the internet looks boring in hindsight today. What it's going to feel like is really good workflow automation yeah, and the people building uh with arcade.
Craig:
are they primarily building uh agents for use within an organization, or are there people building agents for uh for sale to consumers? You know you want an agent to read your and respond to your emails. I mean, yeah, you could go on. Zapier and go through some supposedly simple steps that are not as simple as they sound like they're going to be, or you could just pay a few bucks. Download this agent.
Alex:
Yeah, we see all kinds, we see everything and I'll give you a few examples. So one of our customers is this great product. Everyone should use it. I use it called Shortwave, and they're building an email agent and so you know it helped me organize your inbox. It'll help clear out stuff you don't need to respond to it, help do on subscriptions and help you auto reply to things. You don't have to type as much. It's great, I love it. Shortwave yeah, like shortwave radio. Yeah, totally Look, yeah, totally look it up and big fan.
Alex:
They use us to expand capabilities and so, as you reply to an email, for example, you might want to pull context from the CRM or from a Notion document or your drive, and so we make that easier and more possible for them. We have an agent builder, relevance AI. They're using us to extend their agent building capabilities so that it can all be secure and very consistent and reliable. There's a large financial services organization that's using us for internal productivity, so they're building a Slack bot that integrates to all of the messaging and productivity systems that any individual employee would have. Sneak, which is a big security company. They're using us for a social media app that they built. That they're. They started off in one team. It was so successful they're now rolling it out like wall to wall in the organization and the email. You know this social media agent connects to Twitter and LinkedIn. You know you give it a topic and it will go do the. You know, go prepare everything and research it and and and read your old, your old post to keep it in in your, in your style and tone, and then generate uh the content. Um, so we're doing all of the integrations to the social media platforms.
Alex:
And then, um, there's a very large wealth management uh department at one of the top financial services banks, top Fortune 50. And they are trying to modernize the wealth management system for their clients and they want it to be more agentic, where clients can really ask complex questions about their portfolios and make decisions and have and have it all connect to secure systems because it's very sensitive information. And so we see, we see all kinds Um, I would say the majority of what we see are uh uh, technology companies building a consumer or B2B facing software, um, and then and then financial services. I think, if I were to predict what's coming, I think e-commerce and retail is going to be the next dominant of all. We are part of a partnership with Visa trying to get agentic commerce working. There's a lot of complexity there and I think once things like that start to get released, I think we're going to see a revolution in e-commerce as well.
Craig:
Yeah, yeah. It's fascinating and just going by a sneak, this social media management agent.
Alex:
Is that going to be available to consumers or that's for their internal use and anyone can use that? That's fully open source. It uses Langchain's Landgraf agent framework and Arcade under the hood, so that can be a reference implementation for somebody who wants to do something similar.
Craig:
And that's simple enough for a consumer to use, or is that for companies?
Alex:
I think that one is for developers to turn it into whatever they want to expose. So an intrepid developer could go package that up and release it as a consumer-facing product. But I think it's really designed for developers to repackage.
Craig:
Yeah, have you seen as people are developing these agents? Are there marketplaces of agents or agent stores, you know, as there have been for a lot of other?
Alex:
uh, yeah, there there are a lot of people trying Um, uh, I think what the first iteration of it's looking like are these directories of agents. Um, there are many um and and um, I don't know that I've yet seen one where it's a marketplace where I can legitimately just like pay for things. Uh, I'm sure that's coming. I don't know who will own that category, um, but yeah, I don't. I don't know that an app store has yet come out for agents.
Craig:
Yeah, and maybe it will be the app store. I mean right, and for you guys, when did you start Arcade?
Alex:
We started Arcade a little over a year ago, okay, and it originally started as us trying to build an agent. Um, you know, I was trying to build an agent that could diagnose, uh, production software issues. So, you know, your netflix and for whatever reason, you know, the videos aren't loading fast enough or the servers are crashing for some reason, and traditionally a human has to get involved to go figure out what's going on. And we were building an agent that could go automatically do some of that work. And it was really hard, and the hardest part of it was we. We had a very hard time getting the agent to go connect to all these sensitive systems and be, you know, authorized and authenticated properly and be really consistent in its polls of data, and so we had to reinvent how we were building an agent, and that's ultimately what led us to the insights and the innovation that we pivoted into, which is Arcade. We ultimately took the underlying platform and turned that into its own product.
Craig:
Yeah, and your work with Anthropic and was it Microsoft on MCP. Is that because you guys came out of Okta and are authentication specialists that you got involved? What was the relationship there?
Alex:
Yeah, so MCP is very new as a protocol.
Craig:
Yeah.
Alex:
And Anthropic got a lot of things right, but they didn't get everything right, and so you know how off it's handled was one of those places that was still like something that needed a lot of work and, to Anthropic's credit, instead of just trying to muscle through it themselves, they opened it up to the community to help, you know. They opened up to all the experts, uh, and you know, today, the expert there's a very, it's a very. You know the auth community is not terribly large and and all the experts, uh are either, you know, from Microsoft or Oka alumni, yeah, and and so you know we have, you know, three of the best off people, uh, from octa, uh, on our team, uh, and microsoft, uh, with their intro, formerly known as active directory team, are very expert in this and so, as and so we've been involved as part of the community effort, um, uh, with anthropropic, and we're actually really excited as to where that protocol is going and, in particular, where the auth with it is going. I think it's going to be a really nice developer experience.
Craig:
Is there anything I haven't talked about that you want listeners to know?
Alex:
Yeah, I mean I would say that the biggest ask, if you will, that I have of people, especially either developers themselves or leaders in organizations, is to think past a chatbot. So many people envision an agent and they think chat TPT. But all the biggest places where AI can really have a big impact are really around automating parts of our lives and parts of our work, and many of those use cases are going to look a lot different um than what a chat tpt like interface looks like um, and once people start to realize the art of the possible, that oh wait a second, I can build an agent that can go interact with this and connect to that. There's so much power in what can happen, and so I hope people think bigger, and when they think bigger, I hope they think of us as well.
Craig:
Yeah, and just one last thing on you build an agent, but but I mean, oftentimes in a workflow, uh, it's it's better to to uh make things composable, or or so you may have an agent, rather than having an agent that can do 10 things, break it up so you have 10 agents that work together. Does Arcade account for that? I mean, can you?
Alex:
Yes, yeah, actually. I mean, that's like a whole podcast on its own concept of multi-agent systems. Yeah, we are very big believers in multi-agent systems. Our product is ultimately agnostic, but we're very big believers and when we build agents for ourselves or sample applications, we're increasingly showing off multi-agent systems.
Craig:
Our sponsor for this episode is Xtreme Networks, the company radically improving customer experiences with AI-powered automation for networking. Xtreme is driving the convergence of AI, networking and security to transform the way businesses connect and protect their networks, to deliver faster performance, stronger security and a seamless user experience. Visit extremenetworkscom to learn more. That's extremenetworksallruntogethercom to learn more.
Sonix is the world’s most advanced automated transcription, translation, and subtitling platform. Fast, accurate, and affordable.
Automatically convert your mp3 files to text (txt file), Microsoft Word (docx file), and SubRip Subtitle (srt file) in minutes.
Sonix has many features that you'd love including share transcripts, powerful integrations and APIs, advanced search, automated translation, and easily transcribe your Zoom meetings. Try Sonix for free today.